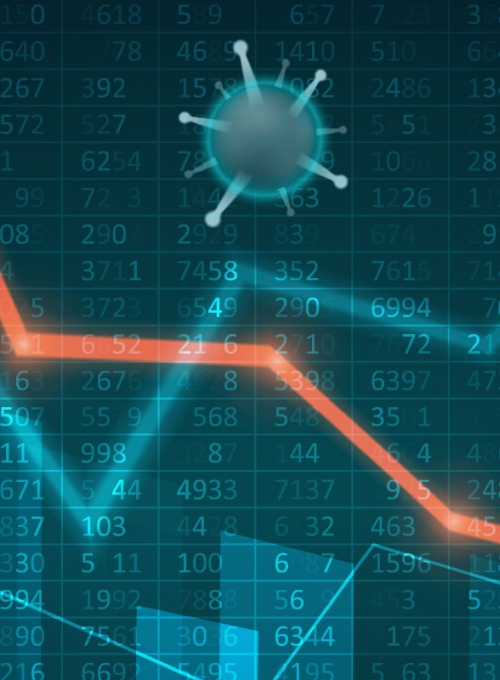
The EPIFORGE 2020 statement
Preferred reporting items for epidemic forecasting and prediction research:
Introduction
The importance of infectious disease epidemic forecasting and prediction research has been underscored across decades of communicable disease outbreaks. Epidemic forecasts are valuable for seasonal pathogens, for example influenza and dengue [1-3], in addition to international health public emergencies and other epidemics such as the Zika, chikungunya, and Ebola virus epidemics [4-9]. Most recently, the Coronarvirus Disease 2019 (COVID-19) pandemic has illustrated the importance of robust, transparent epidemic forecasting and prediction research for risk communication, decision-making, preparedness, and response [10,11]. Arguably, predictions form an essential part of the scientific method itself [12].
Other fields of medical research, such as clinical trials and systematic reviews, have widely used study reporting checklists e.g. the CONSORT and PRISMA guidelines [13]. Such checklists improve the interpretation, evaluation, and reproduction by others scientists and stakeholders, including public health decision-makers,journal editors, and journal reviewers. Indeed, many journals mandate that reporting checklists are completed prior to manuscript submission and publication, which has led to demonstrable improvements in study reporting [14,15]. Although principles for policy-driven communication of models for neglected tropical disease programs have recently been discussed [16], a recent systematic review noted no reporting guidelines exist specifically for epidemic forecasting and prediction research [17]. The need for epidemic forecasting reporting guidelines is underscored by a review of Zika forecasting and prediction research which noted methodological reproducibility, accessibility, and incorporation of uncertainty in these published predictions varied [8].
To address this gap, we developed the EPIFORGE checklist, the first known set of epidemic forecasting reporting guidelines. This checklist was developed through a well-established process for developing guidelines for research reporting, involving a Delphi process and broad consultation with an international panel of infectious disease modelers and model end-users [18,19]. The objectives of these guidelines are to improve the consistency, reproducibility, comparability, and quality of epidemic forecasting reporting. Here we describe our guidelines development process and the resulting checklist. The EPIFORGE checklist is not designed to advise scientists how to perform epidemic forecasting and prediction research, but rather serve as a set of standards to ensure critical aspects of these studies are reported in a standardized way.
Methods
We followed health research reporting guideline development best-practice as outlined in the EQUATOR toolkit, and by Moher et al [18,19]. The EPIFORGE guideline concept was registered at the EQUATOR network [20], and a steering committee (n = 6) formed to develop a guideline development protocol [21]. Members from this steering committee had already identified a case study that prompted the need for EPIFORGE [8], and conducted a systematic review to ensure no epidemic forecasting reporting guideline existed [17]. The EPIFORGE steering committee formulated an initial draft checklist of 20 reporting items during two teleconferences. This draft checklist was the input for an iterative Delphi consensus process as used in other research reporting guidelines [22]. A total of 69 Delphi panelists were invited, and 46 participated in this process. The Delphi panel comprised infectious disease modelers, public health experts who routinely use epidemic forecasts in public health practice, epidemiologists, and biomedical journal editors across several countries (Appendix S1). The candidate panelists were selected by the steering committee to incorporate the perspectives of those who both develop and use models across a range of sectors, including academia, government, and non-government organizations around the globe. Some panelists who were invited further suggested other potential panelists.
During three initial rounds of Delphi consultations via email, panelists graded each checklist item on a scale of1 through 10 (a score of 1 was defined as“not important”, and a score of 10 was defined as “very important”), with an emphasis on voting based on the concept of the item (rather than the wording). Checklist items with a mean score ≥ 8 were retained for the final reporting checklist, items with a mean score <5 were dropped, and items with a mean score 5 –7 were kept for further discussion at a final face-to-face consensus meeting. Additional items were added by Delphi participants during the first two email Delphi rounds. In addition, for each round, panelists were invited to provide comments about the wording of the item, provide a rationale for their vote, and provide citations of evidence to support any new items.
All 46 Delphi panelists were invited to a face-to-face consensus meeting in Baltimore, Maryland (January, 2020). 20 panelists attended either in person or remotely by live video conference. The purpose of the meeting was to discuss intermediately scored items (mean score 5 –7) and vote on them. Those items with a simple majority were included in the final reporting checklist. During this meeting, suggestions about the final wording, and consolidation of similar items, were discussed and documented. The steering committee then drafted a final version of the checklist. This was sent back to all in-person attendees for final comments, and participants had opportunity to ‘pilot test’ the checklist during their own epidemic forecasting activities, including COVID19 forecasting (this invitation for pilot testing yielded no changes to these guidelines). This checklist, along with this elaboration and explanation paper, was then provided to the full Delphi panel for final review and endorsement. During these final reviews, we also requested examples of already published epidemic forecasting papers to illustrate the reporting of specific items.
Results
Table 1 presents the final consensus checklist items, including reporting elements on study goals, data sources, model characteristics and assumptions, model evaluation, and study generalizability. Below we elaborate and explain each item:
A. Overall study description and goals:
Item 1: Study described as a forecast(preferably)or prediction research in at least the title or abstract
While limiting to the terms ‘forecast’, 'forecasting', or 'prediction' may be too restrictive, we believe that limiting the number of terms is important to enable findability (accurate returns on searches) in the literature, and may assist in standardizing nomenclature across the field. For instance, epidemic forecasts may also be referred to as ‘projections’, ‘simulations’, or ‘scenario analyses’ [4,10,23]. While some have previously published definitions of ‘forecasting’ and ‘prediction’ [24], we do not provide a definition of ‘prediction’ or ‘forecasting’ research here in this checklist as we feel this may be too specific.
Item 2: Purpose of study and forecasting targets defined
Clearly identifying the research objectives is a fundamental element of any scientific study, and is a feature of many other research reporting guidelines [13]. Forecasting targets (e.g., two-week-ahead incidence, peak week, observation of at least one case) should be defined in the introduction section, and, ideally, also in the abstract.
Item 3: Methods fully documented
Methods documentation is essential to any scientific study, and follows general best practice for the reporting of other research study types [13]. Forecasting methods should include a full description of the model that enables reproducibility, the method of fitting parameters to data (e.g., maximum likelihood with function if non-standard, Bayesian methods), and –where relevant –underlying epidemic model assumptions (see also Item 8).
Item 4: Identify whether the forecast was performed prospectively, in real-time, and/or retrospectively
This item is necessary for interpreting results of forecasting accuracy, and may aid in determining whether authors were blinded to a hold-out set (out-of-sample set) of data used for any model validations. See also Item 16 for recommendations on time-stamping the results of forecasts.
B. Data description:
Item 5: Origin of input source data explicitly described with references
This item is essential for study reproducibility and is a minimum requirement for any manuscript, even if full study data cannot be publicly shared (see Item 6). For all data types -including laboratory assay, case counts, demographic data, and non-traditional data streams–the authors should include sufficient references to be able to identify the input data, and ideally a persistent and unique identifier that resolves to the (meta)data [9].
Item 6: Source data provided with publication, or reasons as to why this was not possible documented
Provision of source data improves forecast reproducibility. Sharing of source data used in forecasts (e.g., [1]), facilitates other complementary studies, including those which may independently validate forecasts and methods. Limitations on data sharing during epidemics is a known challenge [25]. We are aware of efforts to establish codes of conduct for data sharing during public health emergencies [26], but recognize the wide range of logistical and other barriers to data sharing during outbreaks [25,27]. Therefore, we suggest at a minimum reporting of the reasons for not providing source data with forecast publication. Several major biomedical journals now routinely require authors to provide de-identified data [28]. When data are provided, we recommend inclusion of a data dictionary and/or structured metadata in a standardized format.
Item 7: Input data processing procedures described in detail
This is an important feature for study reproducibility. Pre-processing procedures may include re-coding and imputation of missing observations, identification and management of extreme outliers and influential data points, and functional transformations such as data normalization. Provision of data pre-processing code may also be useful.
C. Model characteristics
Item 8: Statement and description of model type, with model assumptions documented, including references.
This is critical for study reproducibility, and it allows interpretation of model output in the context of any assumptions presented. Describing model parameter values and assumptions, with references, further allows other researchers to use cited parameter values in their own work (after careful consideration), and this may expedite forecasting efforts in a public health emergency. For an ongoing epidemic, if the model makes specific assumptions about current and future interventions and their impact, they need to be stated with appropriate justification. Model types may include mechanistic versus statistical representation of disease transmission, or stochastic versus deterministic models [8]. We do not propose a categorization scheme for model types in these guidelines due to the wide range of model type nomenclature that is often heterogeneously used by modelers. Developing such a schema could be subject of future research.
Item 9: Model code made available, or reason why this is not possible documented
Providing model code improves research reproducibility, especially if accompanied by documentation, and may facilitate the rapid conduct of other studies addressing the same or similar study question(s), especially during a public health emergency. Some forecasting studies already have provided model code during public health emergencies of international concern [6,23]. Infectious disease modelers have also made the point that publication of model code may permit direct comparisons of model performance in real-time by external groups [7]. We emphasize that providing model code is optional, but encouraged. There are valid reasons for why researchers may not be able to provide model code, including intellectual property concerns, or specific concerns about potential mis-use. In that case, we propose a brief justification for why the study’s code is not made available. This may assist in future studies which seek to identify and mitigate barriers to sharing forecast model code during public health emergencies. A clear statement of model code availability will also allow journals to screen submissions for this feature.
D. Model evaluation:
Item 10: Description of model validation, with justification of approach.
Forecast model validation is critical to ensure accuracy of results and usefulness of models, and it also encourages trust in the results and methods by other researchers, journal reviewers, journal editors and end-users. Forecasting research should indicate if cross validation or out-of-sample validation was performed, the data used for validation, how many models were considered at each stage of validation, the time span of validation (with justification), and whether the researchers were blinded to the external validation dataset (e.g., through a prospective design like a forecast challenge or other real-time forecasting exercise) [2,5,29-31].
Item 11: Description of forecast accuracy evaluation method, with justification
Forecast and prediction research studies may include point predictions (e.g. mean number of expected cases) or a full probability distribution of the outcome of interest. It is important that the metric of validation accuracy is both clearly defined and justified, thereby allowing forecast performance to be robustly evaluated and comparison between studies when using the same data.
Item 12: Where possible, compare results to a benchmark or other comparator model, with justification of comparator choice
Benchmark models may include relatively simple models such as autoregression or seasonal averages [32]. These comparisons are important to mitigate the risk of model misspecification and may also provide a "common sense" interpretation of forecast value compared to intuitive bench marks such as an autoregression model with a one week lag-time [33-35]. If there are other published models for the specific forecasting target or type of target that demonstrate significant improvement compared to simpler models, those forecasts should be used as the comparator to the extent possible. Comparison may include formal statistical comparisons with established methods (e.g., Diebold-Mariano tests or permutation tests) [36,37].
Item 13: Description of forecast horizon, with justification of its length
Presenting forecast accuracy with increasing lead-times allows for an evaluation of a forecast’s usefulness over operationally relevant time-scales. We suggest justification of the forecast horizon to avoid inadvertent misrepresentation of model accuracy, and to communicate the inherent limits of forecasts that may break down over longer forecast horizons [32,35].
Item 14: Uncertainty of forecasting results presented and explained
Uncertainty is a fundamental consideration in developing and interpreting epidemic forecasting and prediction research. Uncertainty can arise from parameters, assumptions, model choice, lack of knowledge about the epidemiology of the disease, or variability in the data itself. Qualitative and/or quantitative estimates of uncertainty can be incorporated into forecasting research through using probabilistic forecast methods, uncertainty intervals around point estimates (e.g., 95% credible intervals), sensitivity or scenario analyses, or description of the uncertainty in the model parameters. We recommend that the estimates of uncertainty are clearly described in at least the results,and ideally also referred to in the discussion and the abstract.
E. Translation of results for public health practice, interpretability and generalizability
Item 15: Results briefly summarized in non-technica lterms, including a non-technical interpretation of forecast uncertainty
Adequately reporting and explaining model forecasts is critical for a wide range of readers, including public health decision makers and the media. Forecasts can be misinterpreted, especially when uncertainty is not explicitly and clearly communicated with a broad audience in mind. We propose that a lack of appropriate communication about these inherent caveats in forecasting science may lead to skepticism of forecasting by important end-users (such as decision-makers), the media, and the general public. We recommend a brief non-technical summary of forecasting research results, as already required by several major biomedical journals for a range of research fields [38,39], and including a non-technical interpretation of forecast uncertainty.
Item 16: If results are published as a data object, encourage a time-stamped version number
This reporting recommendation serves multiple purposes. First, it allows searching and aggregating of forecast results by a standardized object nomenclature. Second, it ensures forecasts are truly prospective,when claimed to be so. Third, it permits clear communication of when forecasts are updated (for instance, as parameter estimates are refined or as new data becomes available). We recommend assigning a unique and persistent identifier to the time-stamped and versioned data object, such as a digital object identifier (DOI). This practice could extend to web-based forecasting tools linked to the publication also.
Item 17: Weaknesses of forecast described, including weaknesses specific to data quality and methods
Limitations can include data quality (e.g., heterogeneity in sampling over time and across populations, diagnostic limitations, or case selection bias), parameter uncertainty, model misspecification, or limitations in generalizability. No model is a complete representation of reality, and much can be gleaned about a forecasting model’s utility from knowing its limitations or simplifying assumptions. It is important to note that identifying methodological weaknesses in forecasts does not necessarily mean that they lack credibility. Rather, highlighting such weaknesses may inform data needs, lead to improvements of forecasts, and assist in interpretation of forecast results during public health decision making.
Item 18: If the forecast research is applicable to a specific epidemic, comment on its potential implications and impact for public health action and decision making
When forecasting research is intended to be applicable to a specific outbreak or epidemic, we propose that the potential implications of the forecast for that specific epidemic need to be described, including whether it has a possible impact on public health action or decision-making. Framing the discussion of results in this context is essential for model end-users, and may assist in ensuring that model developers are addressing the right research questions from the outset.
Item 19: If the forecast research is applicable to a specific epidemic, comment on how generalizable it may be across populations
When forecasting research is intended to be applicable to a specific outbreak or epidemic, researchers should describe the generalizability of results between countries, regions, populations,and perhaps even pathogens, together with the rationale for why. A forecast's accuracy or applicability in one setting may not translate to others due to inherent differences in healthcare capacity, population demography, disease ecology, socio-economic factors, and data availability and reliability.
Conclusions
We present the first guidelines for standard reporting of epidemic forecasting research, comprising 19 preferred items in a checklist. We stress that the objectives of these guidelines are intended to improve the epidemic forecasting reporting consistency and reproducibility, as well as comparability and quality. They serve as a set of standards to ensure critical aspects of these studies are adequately reported, and are not intended to advise scientists on how to perform epidemic forecast and prediction research.We noted that our Delphi process also lead to several check-list items which pertain to the translation of forecasting results for public health practice.
The primary target audience of these guidelines are scientists using models to forecast infectious disease epidemics as a means to ensure critical reporting items are included in published manuscripts. While this checklist may also serve as a means of ensuring standardization of infectious disease modeling quality among this group, it is distinct from other structured consensus documents,which have focused on modeling principles or made recommendations for reporting of other types of modeling studies [40-43]. The secondary target audience of these guidelines include model users (e.g.,those in operational public health & policy), journal peer reviewers, journal editors, and epidemiology training programs. We encourage formal endorsement by modeling groups and broad adoption by biomedical journals who already require completion of reporting checklists for manuscript submissions, including clinical trials and systematic reviews [44]. While our guidelines were developed with peer-reviewed published research papers in mind, these could be applied to epidemic forecasting research reported elsewhere.
While the major strength of the EPIFORGE guidelines is the use of a structured Delphi process across a range of stakeholders, this resulted in a number of valuable reporting considerations suggested by the Delphi panel were not included after the consensus process. We noted several items suggested by the Delphi panel that were not ultimately voted in. These covered a range of topics, and may not be applicable to all forecasting and prediction research. We include these items as a supplementary appendix for general consideration in the field of reporting forecasting and prediction research, and these may be reconsidered in future versions of the EPIFORGE reporting guidelines (Appendix S2). While the development process involved broad consultation, we encourage broad and frank feedback and critique. Feedback will be valuable in updating future iterations of these guidelines which are intended to be dynamic and responsive to the ongoing needs of epidemic forecasters and end-users, including those involved in COVID-19 research and response. These guidelines are to be submitted to the EQUATOR Network webpage, in addition to dedicated web pages to facilitate feedback and journal endorsement (https://centerforhealthsecurity.org/epiforge, https://midasnetwork.us/), following examples from other guidelines [14].
Acknowledgement
We appreciate the role of the Outbreak Science and Model Implementation Working Group in developing this initiative, and the Johns Hopkins Center for Health Security for hosting the face-to-face consensus meeting and conducting the electronic Delphi process. We would also like to thank the MIDAS Coordination Center and the National Institutes of General Medical Sciences (NIGMS 1U24GM132013-01) for supporting travel to the face-to-face consensus meeting by members of the Working Group. NGR was supported by the National Institutes of General Medical Sciences (R35GM119582). BMA was supported by Bill & Melinda Gates through the Global Good Fund. RL was funded by a Royal Society Dorothy Hodgkin Fellowship.
Table 1. EPIFORGE 2020 checklist
Section of manuscript | # | Checklist item | Reported on pagea |
Title / Abstract | 1 | Study described as a forecast or prediction in at least the title or abstract | |
Introduction | 2 | Purpose of study and forecasting targets defined | |
Methods | 3 | Methods fully documented | |
Methods | 4 | Identify whether the forecast was performed prospectively, in real-time, and/or retrospectively | |
Methods | 5 | Origin of input source data explicitly described with reference | |
Methods | 6 | Source data made available, or reasons why this was not possible documented | |
Methods | 7 | Input data processing procedures described in detail | |
Methods | 8 | Statement and description of model type, with model assumptions documented with references. | |
Methods | 9 | Model code made available, or reasons why this was not possible documented | |
Methods | 10 | Description of model validation, with justification of approach. | |
Methods | 11 | Description of forecast accuracy evaluation method, with justification | |
Methods | 12 | Where possible, compare model results to a benchmark or other comparator model, with justification of comparator choice | |
Methods | 13 | Description of forecast horizon, and justification of its length | |
Results | 14 | Uncertainty of forecasting results presented and explained | |
Resultsb | 15 | Results briefly summarized in lay terms, including a lay interpretation of forecast uncertainty | |
Results | 16 | If results are published as a data object, encourage a time-stamped version number | |
Discussion | 17 | Limitations of forecast described, including limitations specific to data quality and methods | |
Discussion | 18 | If the research is applicable to a specific epidemic, comment on its potential implications and impact for public health action and decision making | |
Discussion | 19 | If there search is applicable to a specific epidemic, comment on how generalizable it may be across populations |
aThis column refers to where key reporting considerations are included in a manuscript, bA break-out box may be a preferred location
Supplemental material
Appendix S1. Delphi panel members
Appendix S2. Other reporting considerations not included into the final reporting checklist
References
- Lowe R, Stewart-Ibarra AM, Petrova D, Garcia-Diez M, Borbor-Cordova MJ, et al. (2017) Climate services for health: predicting the evolution of the 2016 dengue season in Machala, Ecuador. Lancet Planet Health 1: e142-e151.
- Johansson MA, Apfeldorf KM, Dobson S, Devita J, Buczak AL, et al. (2019) An open challenge to advance probabilistic forecasting for dengue epidemics. Proc Natl Acad Sci U S A 116: 24268-24274.
- McGowan CJ, Biggerstaff M, Johansson M, Apfeldorf KM, Ben-Nun M, et al. (2019) Collaborative efforts to forecast seasonal influenza in the United States, 2015-2016. Sci Rep 9: 683.
- Ahrens KA, Hutcheon JA, Gavin L, Moskosky S (2017) Reducing Unintended Pregnancies as a Strategy to Avert Zika-Related Microcephaly Births in the United States: A Simulation Study. Matern Child Health J 21: 982-987.
- Del Valle SY, McMahon BH, Asher J, Hatchett R, Lega JC, et al. (2018) Summary results of the 2014-2015 DARPA Chikungunya challenge. BMC Infect Dis 18: 245.
- Evans MV, Dallas TA, Han BA, Murdock CC, Drake JM (2017) Data-driven identification of potential Zika virus vectors. Elife 6.
- Funk S, Camacho A, Kucharski AJ, Lowe R, Eggo RM, et al. (2019) Assessing the performance of real-time epidemic forecasts: A case study of Ebola in the Western Area region of Sierra Leone, 2014-15. PLoS Comput Biol 15: e1006785.
- Kobres PY, Chretien JP, Johansson MA, Morgan JJ, Whung PY, et al. (2019) A systematicreview and evaluation of Zika virus forecasting and prediction research during a public health emergency of international concern. PLoS Negl Trop Dis 13: e0007451.
- Rainisch G, Shankar M, Wellman M, Merlin T, Meltzer MI (2015) Regional spread of Ebola virus, West Africa, 2014. Emerg Infect Dis 21: 444-447.
- Moghadas SM, Shoukat A, Fitzpatrick MC, Wells CR, Sah P, et al. (2020) Projecting hospital utilization during the COVID-19 outbreaks in the United States. Proc Natl Acad Sci U S A.
- Alwan NA, Bhopal R, Burgess RA, Colburn T, Cuevas LE, et al. (2020) Evidence informing the UK's COVID-19 public health response must be transparent. Lancet 395: 1036-1037.
- Popper K (2005) The Logic of Scientific Discovery.
- https://www.equator-network.org/library/browse-reporting-guidelines-by-specialty/, accessed April 19 2020.
- http://www.prisma-statement.org/, accessed April 19 2020.
- Hopewell S, Dutton S, Yu LM, Chan AW, Altman DG (2010) The quality of reports of randomised trials in 2000 and 2006: comparative study of articles indexed in PubMed. Bmj 340: c723.
- Behrend MR, Basáñez MG, Hamley JID, Porco TC,Stolk WA, et al. (2020) Modelling for policy: The five principles of the Neglected Tropical Diseases Modelling Consortium. PLoS Negl Trop Dis 14: e0008033.
- Simon Pollett MJ, Matthew Biggerstaff, Lindsay C Morton, Sara L. Bazaco, David M. Brett Major, Anna M. Stewart-Ibarra, Julie A Pavlin, Suzanne Mate, Rachel Sippy, Laurie J Hartman, Nicholas G Reich, Irina Maljkovic Berry, Jean-Paul Chretien, Benjamin M. Althouse, Diane Meyer, Cecile Viboud, Caitlin Rivers (2020) Identification and evaluation of epidemic prediction and forecasting reporting guidelines: a systematic review and a call for action. Epidemics (in-press).
- Moher D, Schulz KF, Simera I, Altman DG (2010) Guidance for developers of health research reporting guidelines. PLoS Med 7: e1000217.
- https://www.equator-network.org/, accessed April 19 2020.
- https://www.equator-network.org/library/reporting-guidelines-under-development/reporting-guidelines-under-development-for-observational-studies/#EPI-FORGE, accessed April 12 2020.
- https://github.com/cmrivers, accessed April 19 2020.
- Chan AW, Tetzlaff JM, Altman DG, Laupacis A, Gotzsche PC, et al. (2013) SPIRIT 2013 statement: defining standard protocol items for clinical trials. Ann Intern Med 158: 200-207.
- Perkins TA, Siraj AS, Ruktanonchai CW, Kraemer MU, Tatem AJ (2016) Model-based projections of Zika virus infections in childbearing women in the Americas. Nat Microbiol 1: 16126.
- Massad E, Burattini MN, Lopez LF, Coutinho FA (2005) Forecasting versus projection models in epidemiology: the case of the SARS epidemics. Med Hypotheses 65: 17-22.
- Chretien JP, Rivers CM, Johansson MA (2016) Make Data Sharing Routine to Prepare for Public Health Emergencies. PLoS Med 13: e1002109.
- https://www.who.int/blueprint/what/norms-standards/GSDDraftCodeConduct_forpublicconsultation-v1.pdf?ua=1, accessed April 19 2020.
- Rivers C, Chretien JP, Riley S, Pavlin JA, Woodward A, et al. (2019) Using "outbreak science" to strengthen the use of models during epidemics. Nat Commun 10: 3102.
- https://journals.plos.org/plosone/s/data-availability, accessed April 19 2020.
- Venkatramanan S, Lewis B, Chen J, Higdon D, Vullikanti A, et al. (2018) Using data-driven agent-based models for forecasting emerging infectious diseases. Epidemics 22: 43-49.
- Lowe R, Barcellos C, Coelho CA, Bailey TC, Coelho GE, et al. (2014) Dengue outlook for the World Cup in Brazil: an early warning model framework driven by real-time seasonal climate forecasts. Lancet Infect Dis 14: 619-626.
- Lowe R, Coelho CA, Barcellos C, Carvalho MS, Catão Rde C, et al. (2016) Evaluating probabilistic dengue risk forecasts from a prototype early warning system for Brazil. Elife 5.
- Reich NG, Brooks LC, Fox SJ, Kandula S, McGowan CJ, et al. (2019) A collaborative multiyear, multimodel assessment of seasonal influenza forecasting in the United States. Proc Natl Acad Sci U S A 116: 3146-3154.
- Pollett S, Boscardin WJ, Azziz-Baumgartner E, Tinoco YO, Soto G, et al. (2017) Evaluating Google Flu Trends in Latin America: Important Lessons for the Next Phase of Digital Disease Detection. Clin Infect Dis 64: 34-41.
- Olson DR, Konty KJ, Paladini M, Viboud C, Simonsen L (2013) Reassessing Google Flu Trends data for detection of seasonal and pandemic influenza: a comparative epidemiological study at three geographic scales. PLoS Comput Biol 9: e1003256.
- McGough SF, Brownstein JS, Hawkins JB, Santillana M (2017) ForecastingZika Incidence in the 2016 Latin America Outbreak Combining Traditional Disease Surveillance with Search, Social Media, and News Report Data. PLoS Negl Trop Dis 11: e0005295.
- https://www.tandfonline.com/doi/abs/10.1198/073500102753410444, accessed April 19 2020.
- Ray EL, Reich NG (2018) Prediction of infectious disease epidemics via weighted density ensembles. PLoS Comput Biol 14: e1005910.
- Shailes S (2017) Something for everyone. Elife 6.
- http://thelancet.com/pb/assets/raw/Lancet/authors/tlrm-info-for-authors.pdf, accessed June 19, 2020.
- Caro JJ, Briggs AH, Siebert U, Kuntz KM (2012) Modeling good research practices--overview: a report of the ISPOR-SMDM Modeling Good Research Practices Task Force--1. Value Health 15: 796-803.
- Briggs AH, Weinstein MC, Fenwick EA, Karnon J, Sculpher MJ, et al. (2012) Model parameter estimation and uncertainty: a report of the ISPOR-SMDM Modeling Good Research Practices Task Force--6. Value Health 15: 835-842.
- Dahabreh IJ, Trikalinos TA, Balk EM, Wong JB (2008) AHRQ Methods for Effective Health CareGuidance for the Conduct and Reporting of Modeling and Simulation Studies in the Context of Health Technology Assessment. Methods Guide for Effectiveness and Comparative Effectiveness Reviews. Rockville (MD): Agency for Healthcare Research and Quality (US).
- Pitman R, Fisman D, Zaric GS,Postma M, Kretzschmar M, et al. (2012) Dynamic transmission modeling: a report of the ISPOR-SMDM Modeling Good Research Practices Task Force Working Group-5. Med Decis Making 32: 712-721.
- https://journals.plos.org/plosmedicine/s/submission-guidelines#loc-guidelines-for-specific-study-types, accessed April 23 2020